일 | 월 | 화 | 수 | 목 | 금 | 토 |
---|---|---|---|---|---|---|
1 | 2 | 3 | 4 | 5 | ||
6 | 7 | 8 | 9 | 10 | 11 | 12 |
13 | 14 | 15 | 16 | 17 | 18 | 19 |
20 | 21 | 22 | 23 | 24 | 25 | 26 |
27 | 28 | 29 | 30 |
- pyplot
- XGBoost
- 머신러닝
- Golang
- 지니계수
- bar
- Heatmap
- matplotlib
- GINI
- ml
- sklearn
- decisiontree
- Python
- confusion matrix
- gini coefficient
- scatter
- Today
- Total
목록pyplot (4)
Passion, Grace & Fire.
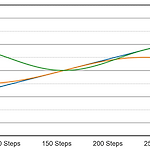
tick에 배정되는 tick label의 문자열 변경은 get_yticklabels() / set_yticklabels() 함수를 이용하여 할 수 있다. yticks = ax.get_yticks() ax.set_yticks(yticks) yticklabels = ax.get_yticklabels() ax.set_yticklabels([f'{y:.0f} Value' for y in yticks], fontdict={"fontsize":16, "color":"black", "ha":"right", "va":"center"}) pyplot 그래프 출력 시 기존 tick과는 별도로 보조 tick을 출력할 수 있다. major/minor tick의 간격은 set_major_locator()/set_minor_lo..
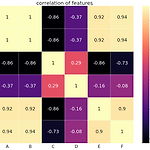
heatmap은 색상표에 기반하여 데이터를 시각화하는 그래픽 표현이다. 머신러닝 분야에서는 여러개의 feature에 대해 feature 간 연관성(상관관계)를 시각화하려고 할 때 많이 사용된다. 상관관계가 높은 feature들은 줄일 수 있기 때문에 성능개선에 도움이 된다. feature마다의 상관관계(correlation)를 heatmap으로 표현하는 예제 코드이다. 먼저 데이터를 준비한다. import pandas as pd import matplotlib.pyplot as plt import seaborn as sns data = {'A' : [1, 0, 3, 2, 4, 5, 8], 'B' : [1, 0, 3, 2, 4, 5, 8], 'C' : [7, 6, 3, 4, 5, 2, 1], 'D' : [..
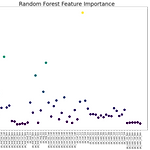
데이터의 분포를 표현할 때 사용할 수 있다. values = [0.01686434542269142, 0.011053805247045436, 0.036219170535060755, 0.019470566624956092, 0.07861381065229754, 0.019871398042689628, 0.02209323746126317, 0.004198705181889577, 0.003607748989459628, 0.0003743181308508165, 0.000658587568488969, 0.0013468714834950239, 0.0006631273358967262, 0.002396248137555366, 0.02559893166563769, 0.013920353817181067, 0.0569721290..
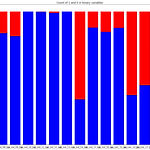
bar plot의 경우 bottom에 기존에 그린 데이터를 넘겨 bar를 쌓을 수 있다. import matplotlib.pyplot as plt zero_list = [360852, 442223, 497644, 484917, 594990, 594205, 589594, 594648, 201882, 523143, 503879, 522342, 221514, 265356, 424278, 387469, 503955] one_list = [234360, 152989, 97568, 110295, 222, 1007, 5618, 564, 393330, 72069, 91333, 72870, 373698, 329856, 170934, 207743, 91257] features = ['ps_ind_06_bin', 'ps_i..